When you hear the word chaos, what comes to mind? Do you think of randomness, of messiness and unpredictability? What about market crashes and war? When I hear the word chaos, I think of patterns and I think of beauty.
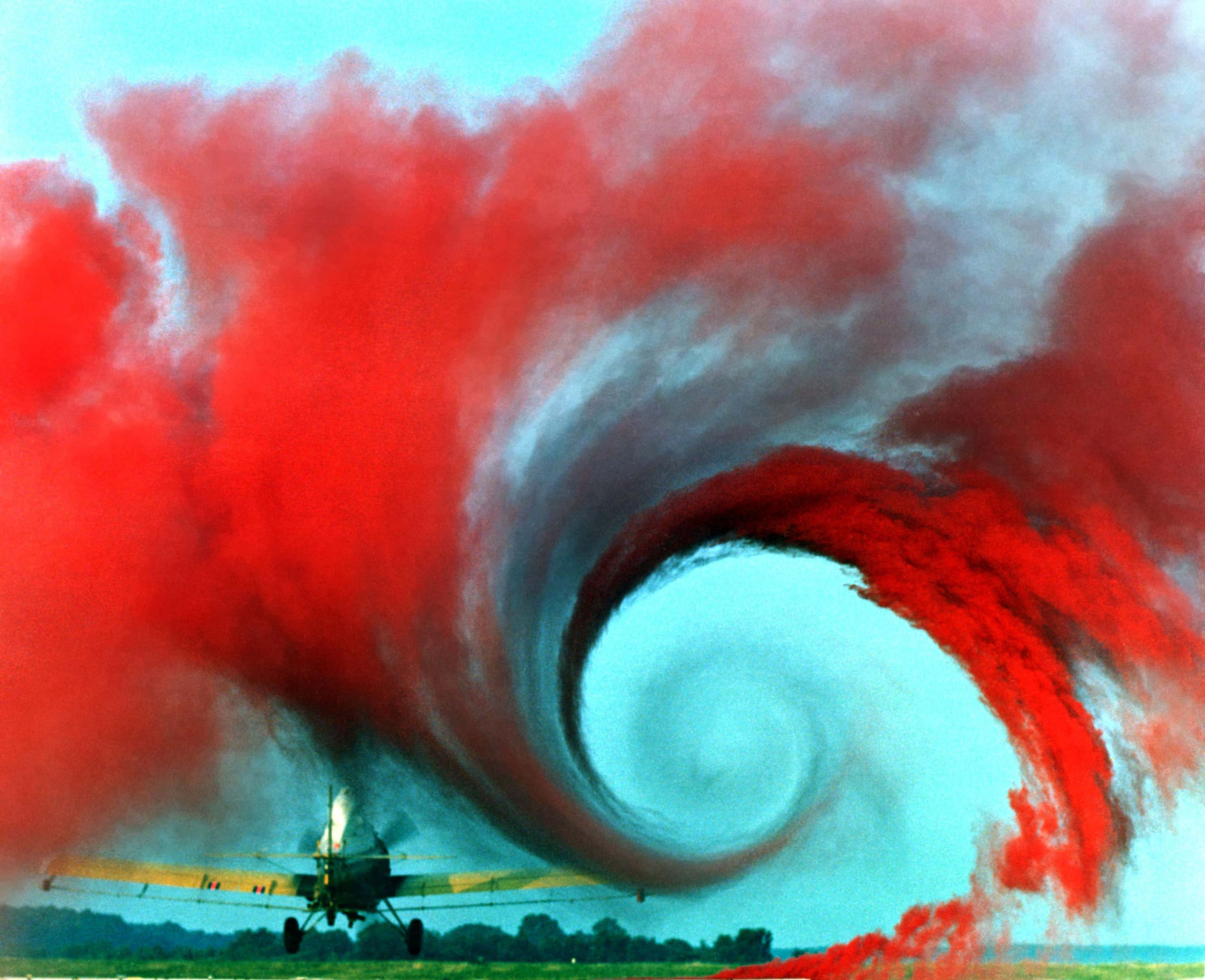
A study of turbulence at the Wallops Flight Facility. Red smoke is released from the ground and gets caught in a vortex caused by the airplane wing cutting through the air.
NASA Langley Research Center, public domain
For a long time, physicists thought that we were going to be able to tell the future with physics. Once we knew all the rules (F = ma, for example), we would be able to predict the next storm or earthquake as accurately as we predict a solar eclipse. But that was before we understood chaos. Chaos prevents us from making useful predictions about certain physics phenomena through something called sensitivity. There are some systems in which the future is extremely sensitive to what is happening right now so that any small change in current conditions has huge repercussions down the road. These systems are called chaotic. The behavior of these systems could theoretically be predicted, which differentiates them from truly random systems, but it is impossible in practice. How can you make a reliable prediction when tiny effects (sometimes smaller than we can measure or simulate) can drastically change the eventual outcome? Several movies have been made to dramatize the effects of chaos on human lives. In The Butterfly Effect and Sliding Doors, seemingly small and insignificant changes and choices lead to significantly different outcomes for the characters in different timelines. In fact, almost any story with time travel plays with the premise: a small change now might lead to a huge change later on.
Like many areas of mathematics, the field of chaos has an unusual origin story. Back in the 1880s King Oscar II of Sweden wanted to celebrate his 60th birthday in style. So he did the obvious thing: he held a mathematics competition. He chose the three-body problem, a classic problem in celestial mechanics, which asks to describe the motion of three celestial bodies which move in each other’s gravity, such as a planet moving close to two stars. The two-body problem, which asks to describe the motion of just two celestial bodies, had already been solved: the two bodies orbit each other in perfect ellipses. If you know their motion at one moment, then you can predict it for eternity.
But since the days of Sir Isaac Newton, the greatest mathematicians in the world had tried and failed to produce a similar result for the three-body problem. Just adding one more celestial body to the equation made the problem fiendishly difficult. But mathematician Henri Poincaré was not one to shy away from difficult problems, and so he accepted the challenge of the Swedish King . Using only pen and paper mathematics, Poincaré made great progress on the analysis of the three-body problem and, though he failed to solve the problem, he won the king’s prize. But later he realized there was a mistake in his submission, and in correcting the mistake he discovered something shocking: if he slightly perturbed the position of one of the planets, it would lead to a drastically different trajectory over time. The concepts of sensitivity and chaos were born .
If you look around, you can see chaos manifest itself in everyday life. Have you ever noticed that every cloud in the sky is unique? Take a look—each cloud has a slightly different shape. That’s because the growth of clouds is chaotic: each cloud experiences tiny differences in temperature, humidity, and wind speed as it grows, leading to differences that ultimately become big enough to be visible from thousands of feet away. Chaos is also why a double pendulum will never show the same motion twice. A human hand isn’t steady enough to start it in exactly the same place twice, so we can’t predict or duplicate the behavior. Here’s a video of this phenomenon:
Two double pendula swing side by side. Although released from very similar points, the pendula show drastically different motion.
Coolphysicsvideos
I study chaos where fluids, such as air or water, move over a surface. Think of the flow of air around a plane wing or the flow of water along the hull of a ship. Instead of moving uniformly in a certain direction, sometimes the fluid gets tripped up and starts swirling. One reason this can happen is if rough parts of the surface ‘catch’ the fluid. Once the fluid trips up, it enters a messy swirling state called “turbulence”, and it is not a coincidence if you associate that word with bumpiness on flights. The turbulence that you might feel on a plane is made of big versions of these swirls, generated as air moves past the Earth’s surface. When the plane flies through these swirls, it gets jostled around, leading to bumps for the passengers. Tiny versions of these air swirls are also caused by the airplane itself, increasing friction and transportation fuel costs. Over 50% of drag on commercial airplanes comes from turbulence kicked up by the surface of the plane ). These tiny swirls also fill water and oil pipelines, increasing required pumping power . Like clouds, you’ll never see exactly the same swirl shape twice. And like a double pendulum, you’ll never see exactly the same swirl motion twice. Here’s a beautiful video of turbulence past a flat surface:
A flat plate is dragged through a fluid. The fluid is kicked up as the plate moves past. Dye is injected to make this visible.
Lee, Kwon, Hutchins, and Monty, University of Melbourne
But even with the uniqueness of each cloud’s shape, you can still look up in the sky and recognize a cloud. And in fact, you might be able to do even better than “cloud”. There are differents types of clouds, such as cumulus and cumulonimbus, and you may be able to categorize the cloud as such. You might even be able to predict whether a cloud on the horizon will lead to rain. This human ability is called pattern recognition—the ability to generalize and group objects even if the objects aren’t exactly the same. Many clouds might be sitting at approximately the same height in the atmosphere, they might be approximately the same size, and the definition of their edges against the sky might be approximately the same. By generalizing a little and identifying common patterns, you can make important predictions about what will happen next, even in systems that are chaotic.
I search for patterns of swirls in turbulence in much the same way that a weather forecaster might search for clouds in the sky. And while we as humans are very good at generalizing and identifying patterns, computers sometimes struggle when two things aren’t exactly the same. I teach computers what types of generalizations we can make about the shape of swirls so that we can categorize swirls automatically. This is part of a larger effort in the scientific community to develop automatic pattern recognition. A slight distinction exists, however, between pattern recognition for physics problems and pattern recognition for certain technologies such as computer vision. Self-driving cars use computer vision: the car needs to be able to distinguish a telephone pole from a person, for example. For a car to function, it does not matter how or why the computer can distinguish between people and telephone poles, and so software engineers allow the computer to make its own logic that gets hidden in its complex and organic learning process. As physicists, however, we do not only want to distinguish between different patterns, we also want to understand why each pattern is important to the structure and motion of turbulence. We therefore use slightly different tactics to identify patterns. My advisor, Professor Beverley McKeon, and I have recently proposed a model for the average shape of a particular pattern in turbulence. We show that our model matches the pattern’s statistical signature, and matches its physical shape . The model is derived from the Navier-Stokes equations (Newton’s second law for fluid dynamics), which describe the physics of the problem. That the model comes from the fundamental equations allows for direct interpretation of the importance of the pattern within the physics of fluid flows. I also study how turbulent swirls affect other swirls nearby. In a double pendulum, each segment of the pendulum pulls at its partnered segment and modifies its motion. In turbulence, each swirl can affect the other swirls too—it can pull and push, leading to complex motion. And it turns out that by generalizing in the right ways, we can identify patterns in the interactions between swirls too. Some of our recent work identified and modeled the interaction of particular patterns, and showed that these interactions affect how heat is moved around in air .
By categorizing the types of swirls that we see and understanding how they affect one another, we move towards being able to predict what a turbulent flow will do next. Prediction has many important practical applications. Efficient prediction could allow us to design better planes and ships to decrease fuel usage, a huge environmental problem. And someday, it could allow us to have much more maneuverable, stable planes that can adjust to gusts so that swirls in the atmosphere don’t cause bumps for passengers. By sensing and immediately reacting to flow conditions, maybe we could develop aircraft that modify their shape and motion—like a bird bending its wing—to account for the swirls in the atmosphere, leading to smoother flights. Seeking patterns in chaos is a fascinating and challenging pursuit. Finding these patterns has huge potential for real-world applications. But there is another reason why I study chaos. Just go back and watch the video of the double pendulum, or the vortex swirls. Go outside and look at the clouds. No other system, no other moment in time will ever look quite like that. Every moment is utterly new. The joy of my work, and arguably my life, lies in the constant uniqueness of chaos.
I would like to acknowledge my advisor, Professor Beverley McKeon, who guided me in the development of this research, and my funding sources, the National Defense Science and Engineering Graduate (NDSEG) Fellowship from the Department of Defense, and two Air Force grants #FA9550-12-0060 and #FA9550-16-1-0361. Thanks also to the editor, Jeremy Bernstein, for contributing to the section on history of chaos.